Infra
Make machine learning more reproducible and easier to manage for the broader community by building logging tools and recommending approaches for tracking and operating machine learning systems.
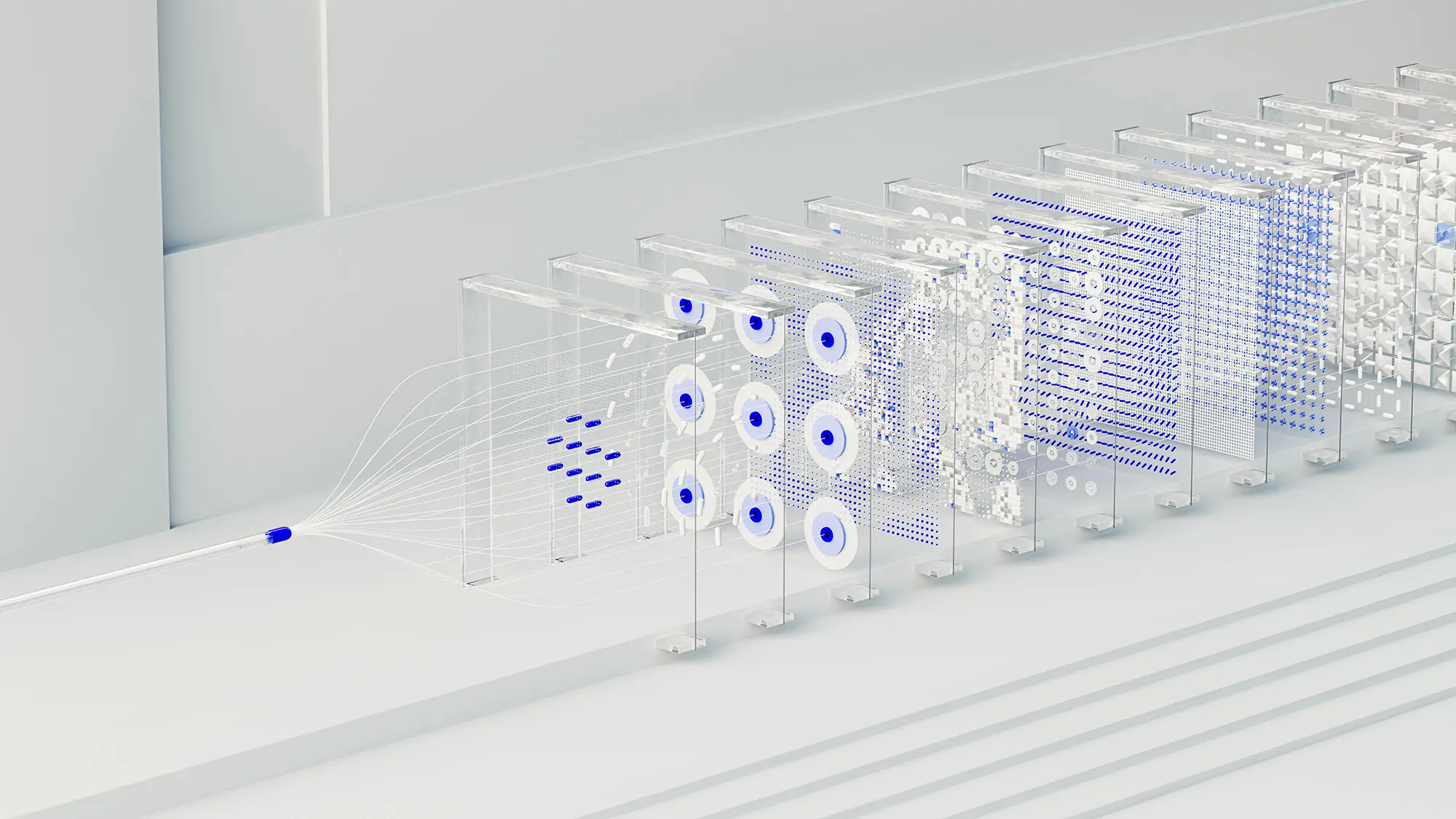
Purpose
Across MLCommons projects, we strive to simplify user experience by providing a unified set of tools. Centralized logging tools are especially critical because they simplify rules compliance and ensure that all vendor submissions for MLPerf benchmarks are easy to debug and capture the relevant ML system details.
The Infra working group strives to improve reproducibility of results and automation of documentation about results. By understanding system-level specs and increasing reproducibility, we can start to build a more detailed matrix of performance-impacting factors. By improving automation, we can improve user experience and verify that each vendor submission includes requisite information.
Deliverables
- Logging and reporting tools for MLCommons projects
- Logging metrics and format
- Definition and examples of system specs
- Roadmap for unified logging tools across MLCommons projects aligned with inference, training, best practices, etc. roadmaps
- Best practices for MLPerf training and inference result reproducibility
Meeting Schedule
Tuesday January 28, 2025 Weekly – 10:35 – 11:25 Pacific Time
How to Join and Access Infra Working Group Resources
The Infra working group is limited exclusively to MLCommons members and affiliates. If you are not already a member or affiliate or part of a member affiliate company, you can learn more about MLCommons membership here.
To sign up for the group mailing list, receive the meeting invite, and access shared documents and meeting minutes:
- Fill out our subscription form and indicate that you’d like to join the Infra Working Group.
- Associate a Google account with your organizational email address.
- Once your request to join the Infra Working Group is approved, you’ll be able to access the Benchmark Infra folder in the Members Google Drive.
To engage in group discussions, join the group’s channels on the MLCommons Discord server.
Infra Working Group Chairs
To contact all Infra working group chairs email [email protected].