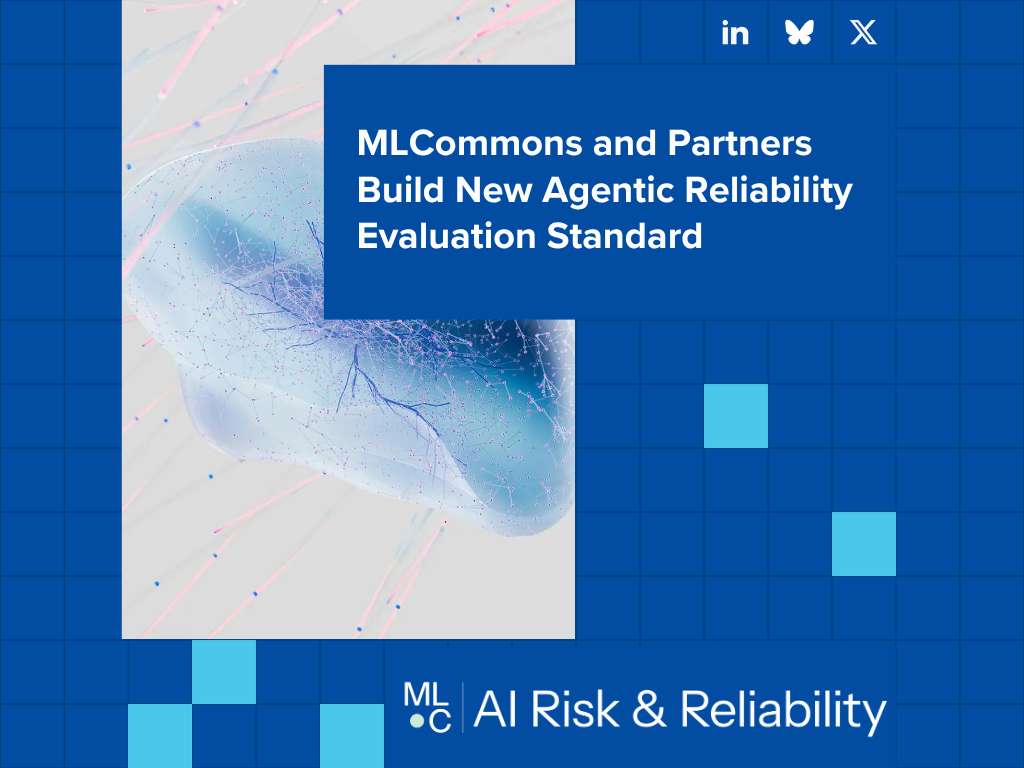
AI Risk & Reliability
Support community development of AI risk and reliability tests and organize definition of research- and industry-standard AI safety benchmarks based on those tests.
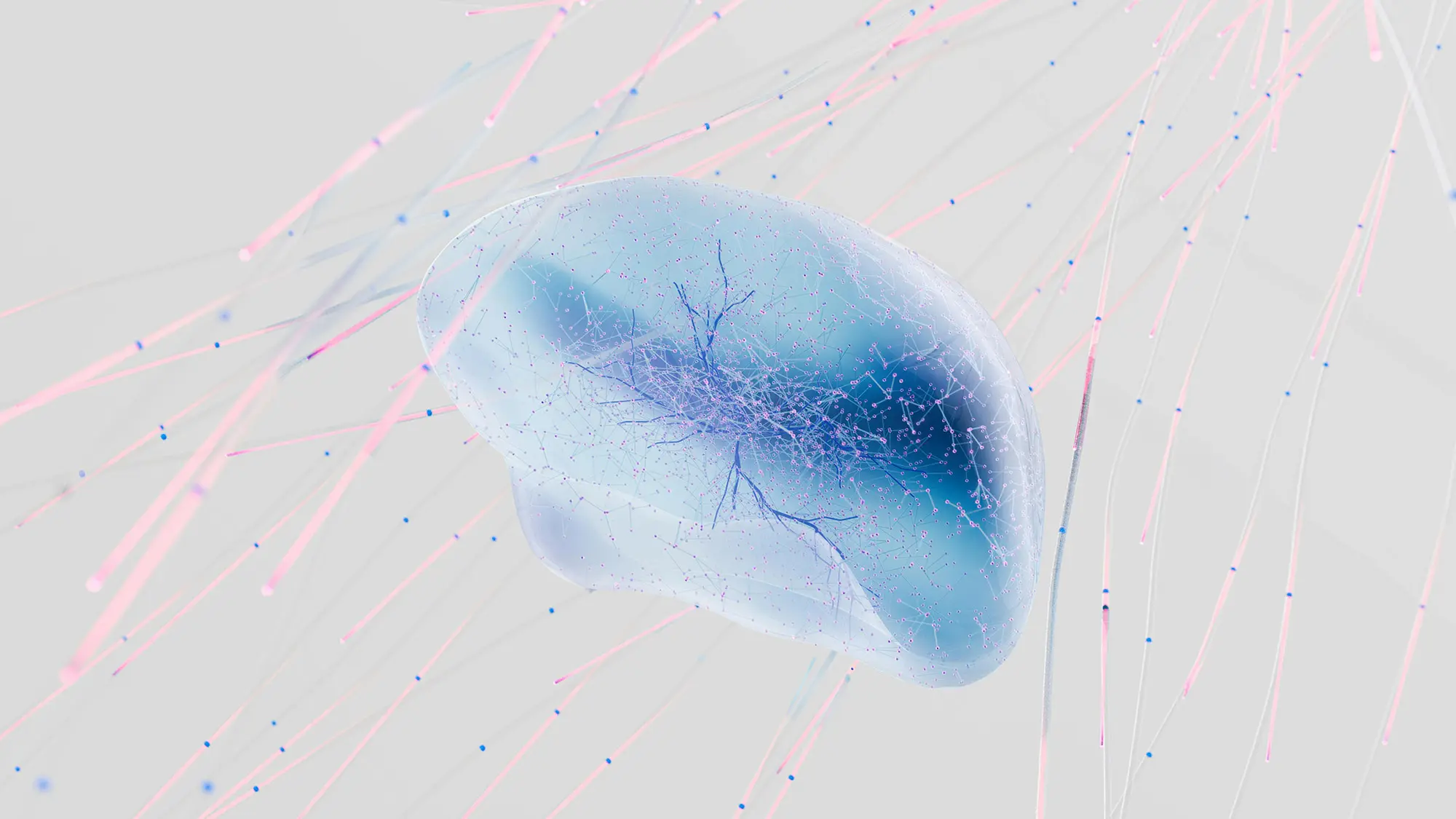
Purpose
Our goal is for these benchmarks to guide responsible development, support consumer / purchase decision making, and enable technically sound and risk-based policy negotiation.
Deliverables
We are a community based effort and always welcome new members. There is no previous experience or education required to join as a volunteer. Specifically, the working group has the following four major tasks:
- Tests: Curate a pool of safety tests from diverse sources, including facilitating the development of better tests and testing methodologies.
- Benchmarks: Define benchmarks for specific AI use-cases, each of which uses a subset of the tests and summarizes the results in a way that enables decision making by non-experts.
- Platform: Develop a community platform for safety testing of AI systems that supports registration of tests, definition of benchmarks, testing of AI systems, management of test results, and viewing of benchmark scores.
- Governance: Define a set of principles and policies and initiate a broad multi-stakeholder process to ensure trustworthy decision making.
Meeting Schedule
Friday January 17, 2025 Weekly – 08:30 – 09:30 Pacific Time
AI Risk & Reliability Working Group Projects
How to Join and Access Resources
To sign up for the group mailing list and receive the meeting invite:
- Fill out our subscription form and indicate that you’d like to join the Medical Working Group.
- Associate a Google account with your organizational email address.
- Once your request to join the AI Risk & Reliability working group is approved, you’ll be able to access the AI Risk & Reliability folder in the Public Google Drive.
To access the GitHub repositories (public):
- If you want to contribute code, please submit your GitHub username to our subscription form.
- Visit the GitHub repositories:
AI Risk & Reliability Working Group Workstreams and Leads
- Multimodal, workstream leads: Ken Fricklas and Lora Aroyo
- Scaling and Analytics, workstream leads: James Ezick and Heather Frase
- Agentic, workstream lead: Fazl Barez
- Adoption, workstream lead: Mike Kuniavsky
AI Risk & Reliability Working Group Chairs
To contact all AI Risk & Reliability working group chairs email [email protected].
Joaquin Vanschoren
Percy Liang
Peter Mattson
Questions?
Reach out to us at [email protected]