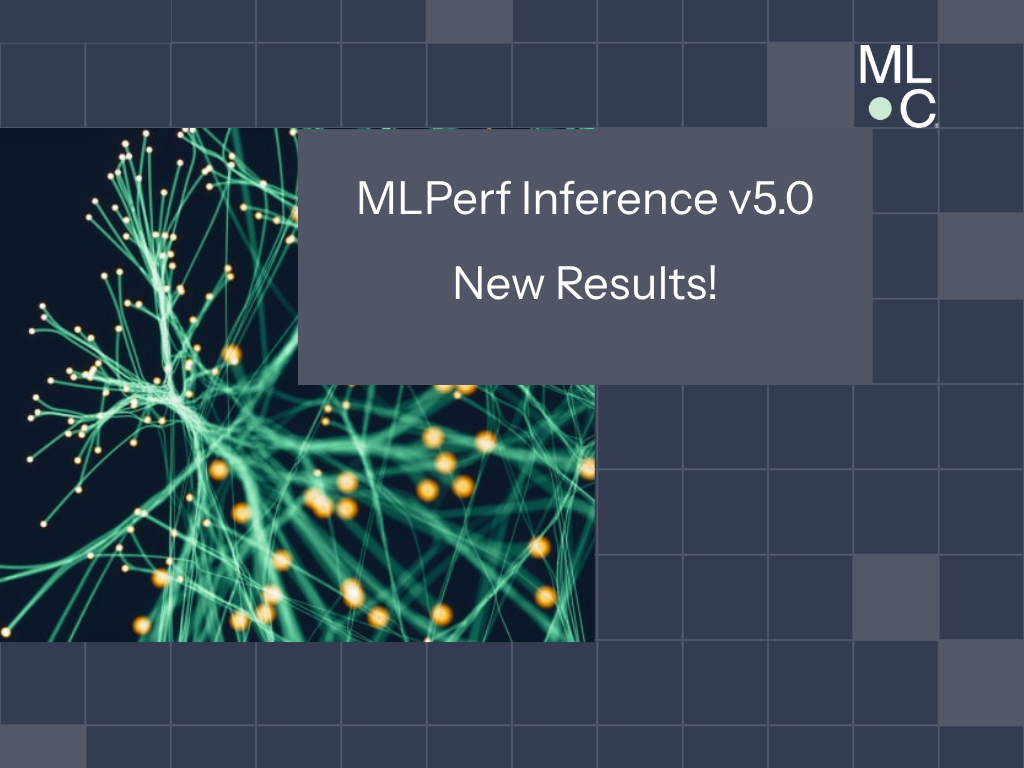
MLPerf Inference
Create a set of fair and representative inference benchmarks.
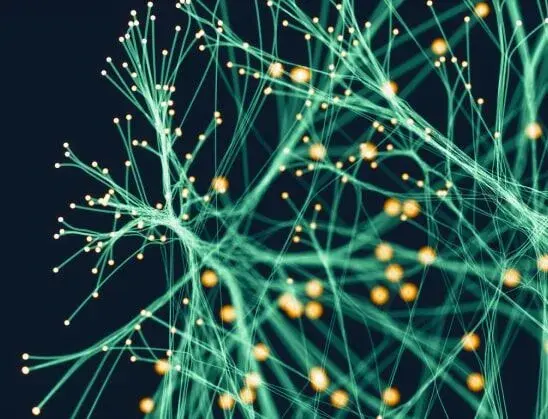
Purpose
Machine-learning (ML) hardware and software system demand is burgeoning. Driven by ML applications, the number of different ML inference systems has exploded. Over 100 organizations are building ML inference chips, and the systems that incorporate existing models span at least three orders of magnitude in power consumption and five orders of magnitude in performance; they range from embedded devices to data-center solutions. Fueling the hardware are a dozen or more software frameworks and libraries. The myriad combinations of ML hardware and ML software make assessing ML-system performance in an architecture-neutral, representative, and reproducible manner challenging. There is a clear need for industry-wide standard ML benchmarking and evaluation criteria. MLPerf Inference answers that call.
Deliverables
- Inference benchmark rules and definitions
- Inference benchmark reference software
- Inference benchmark submission rules
- Inference benchmark roadmap
- Publish inference benchmark results every ~6 months
Meeting Schedule
Tuesday July 15, 2025 – 08:35 – 10:00 Pacific Time
Results Publication
July 25, 2025 Friday
How to Join and Access MLPerf Inference Resources
This group is limited exclusively to MLCommons members and affiliates. If you are not already a member, affiliate or part of a member or affiliate company, you can learn more about MLCommons membership here.
To sign up for the group mailing list, receive the meeting invite, and access shared documents and meeting minutes:
- Fill out our subscription form and indicate that you’d like to join the MLPerf Inference Working Group.
- Associate a Google account with your organizational email address.
- Once your request to join the Inference Working Group is approved, you’ll be able to access the Inference folder in the Members Google Drive.
To engage in group discussions, join the working group’s channels on the MLCommons Discord server.
To access the GitHub repository (public):
- If you want to contribute code, please submit your GitHub ID to our subscription form.
- Visit the GitHub repository.
Inference Working Group Chairs
To contact all MLPerf Inference working group chairs email [email protected].