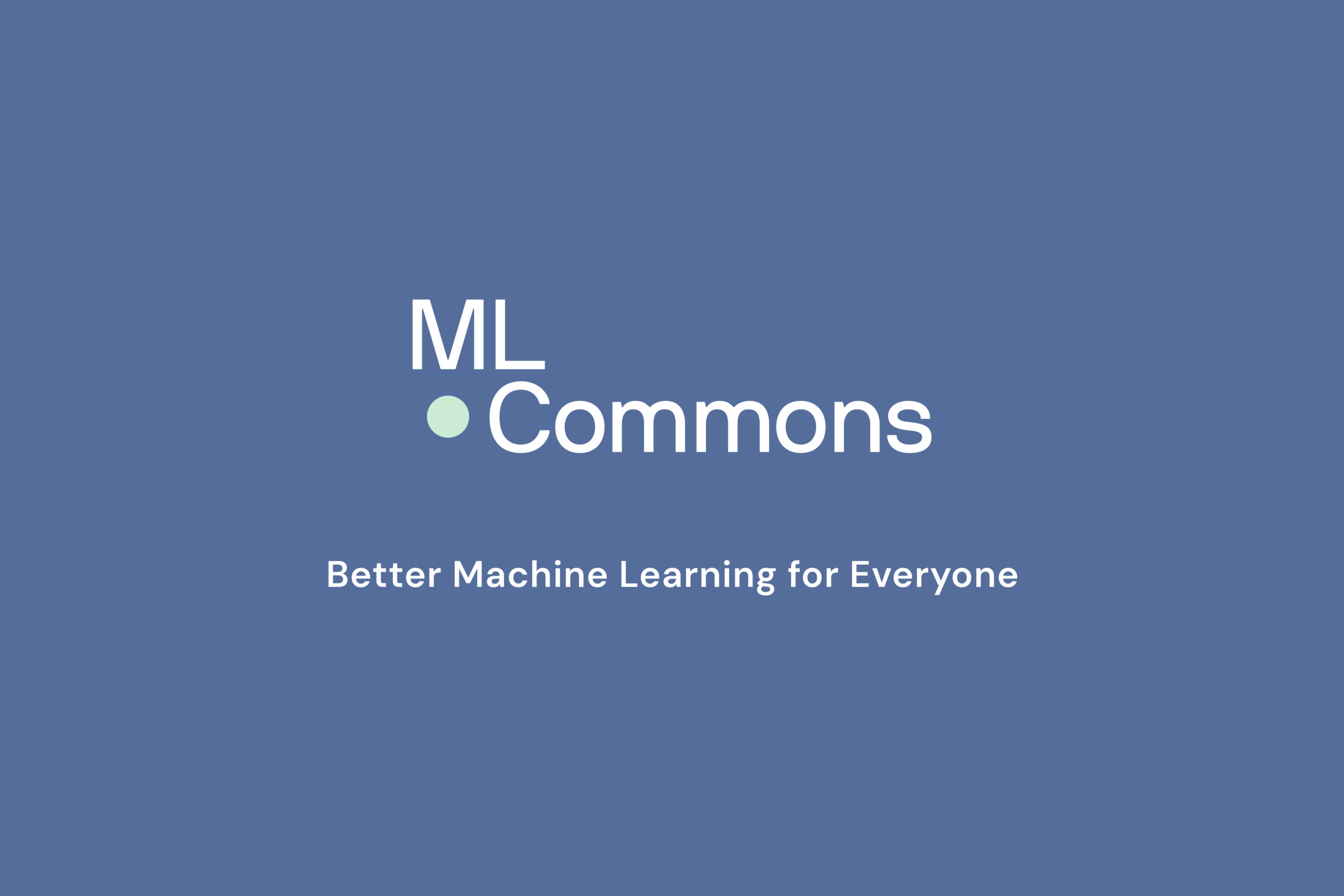
Chakra
Advance performance benchmarking and co-design using standardized execution traces.
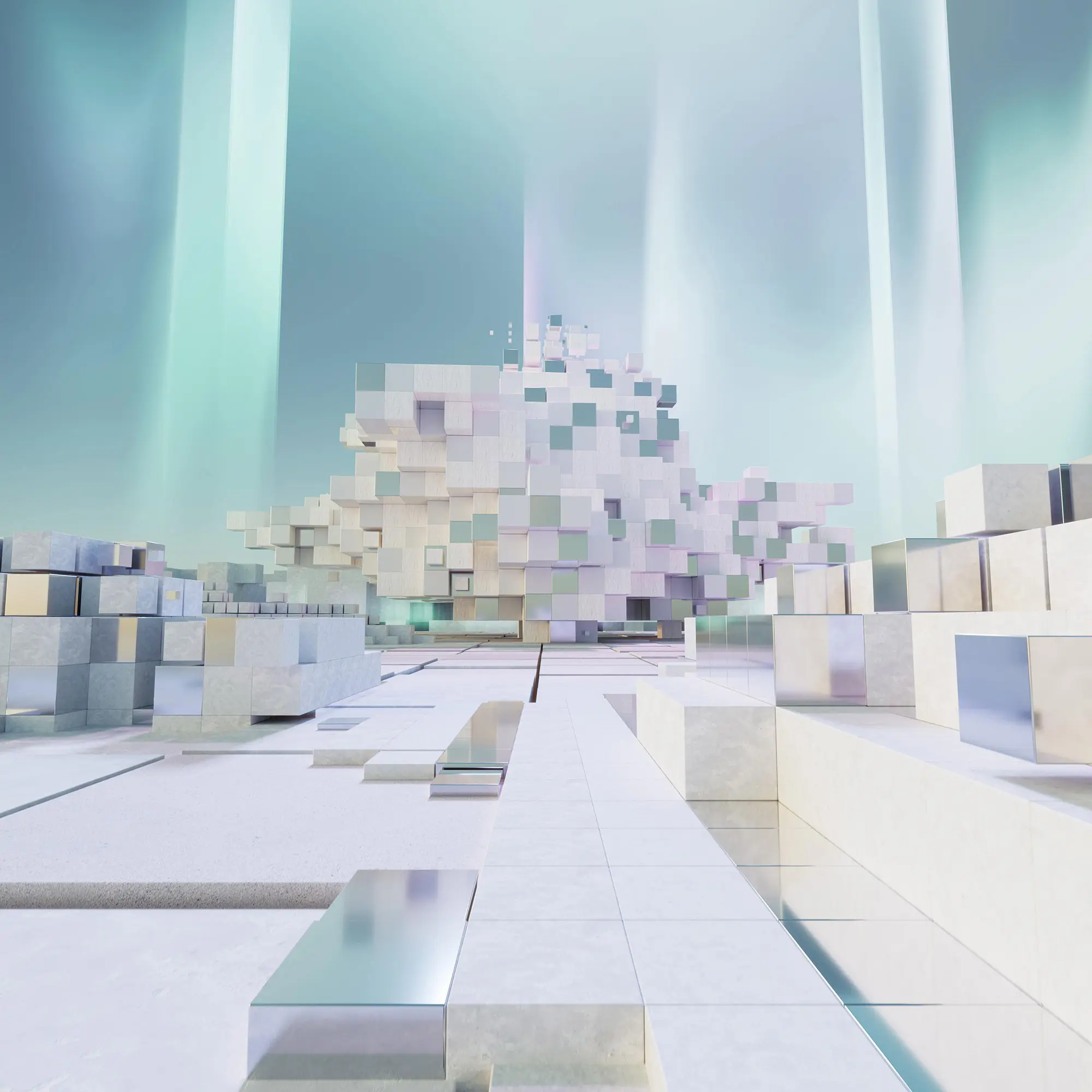
Purpose
Benchmarking and co-design are essential for driving optimization and innovation around AI models, software, and next-generation hardware. Full-stack benchmarks (such as MLPerf) play a crucial role in enabling fair comparisons across different software and hardware stacks on current systems. However, the fast pace of AI innovation demands a more agile methodology for reproduction of workload behavior in current production systems and extension to future AI SW/HW system co-design. Examples include:
- Enable production teams in cloud/hyperscalers and/or vendors quickly reproduce a bug or performance regression based on behavior observed in production
- Project workload behavior for co-designing future AI systems, either for use cases internal to cloud/hyperscalers, sharing with vendors (with or without NDAs), and/or sharing broadly across industry/academia in an open manner.
Existing benchmarking methodologies fall short in addressing these requirements. Creating a small stand-alone reproducer is time-consuming and usually requires non-trivial effort. Furthermore, for external use cases it is critical we are able to accomplish the above without sharing proprietary code/model details. The challenge emanates (in part) from (i) lack of standard mechanisms to define and share workload behavior without sharing the actual code/infrastructure, and (ii) lack of interpretability or comparison across a wide range of proprietary simulation and emulation tools preferred by different vendors.
We are developing Chakra: an open and interoperable graph-based representation of AI/ML workloads focused on enabling and accelerating AI SW/HW co-design. Chakra execution traces represent key operations, such as compute, memory, and communication, data and control dependencies, timing, and resource constraints. Additionally, Chakra includes a complementary set of tools and capabilities to enable the collection, analysis, generation, and adoption of Chakra ETs by a broad range of simulators, emulators, and replay tools.
The Chakra working group is working on the following components:
- Schema standardization
- Execution trace collection – PyTorch, TF/XLA, etc.
- Chakra trace synthesis using ML models (for trace obfuscation and future workload projection)
- Support tools, e.g. analyzers, visualization, etc.
- Downstream tools enablement, e.g. simulators, emulators, replay
- Benchmark suite development and metrics
Deliverables
- Defining the Chakra schema
- Support for collecting Chakra traces in commonly used AI frameworks
- Benchmark definition, methodology, and scoring representing different classes of AI workloads
- Building consensus on tasks, models, rules, first submissions, and leaderboard
Meeting Schedule
Monday May 19, 2025 Weekly – 11:05 – 12:00 Pacific Time
How to Join and Access Chakra Resources
To sign up for the group mailing list, receive the meeting invite, and access shared documents and meeting minutes:
- Fill out our subscription form and indicate that you’d like to join the Medical Working Group.
- Associate a Google account with your organizational email address.
- Once your request to join the Chakra Working Group is approved, you’ll be able to access the Chakra folder in the Public Google Drive.
To engage in working group discussions, join the group’s channels on the MLCommons Discord server.
To access the GitHub repositories (public):
- If you want to contribute code, please submit your GitHub ID to our subscription form.
- Visit the GitHub repository.
Chakra Working Group Chairs
Chairs
To contact all Chakra working group chairs email [email protected].