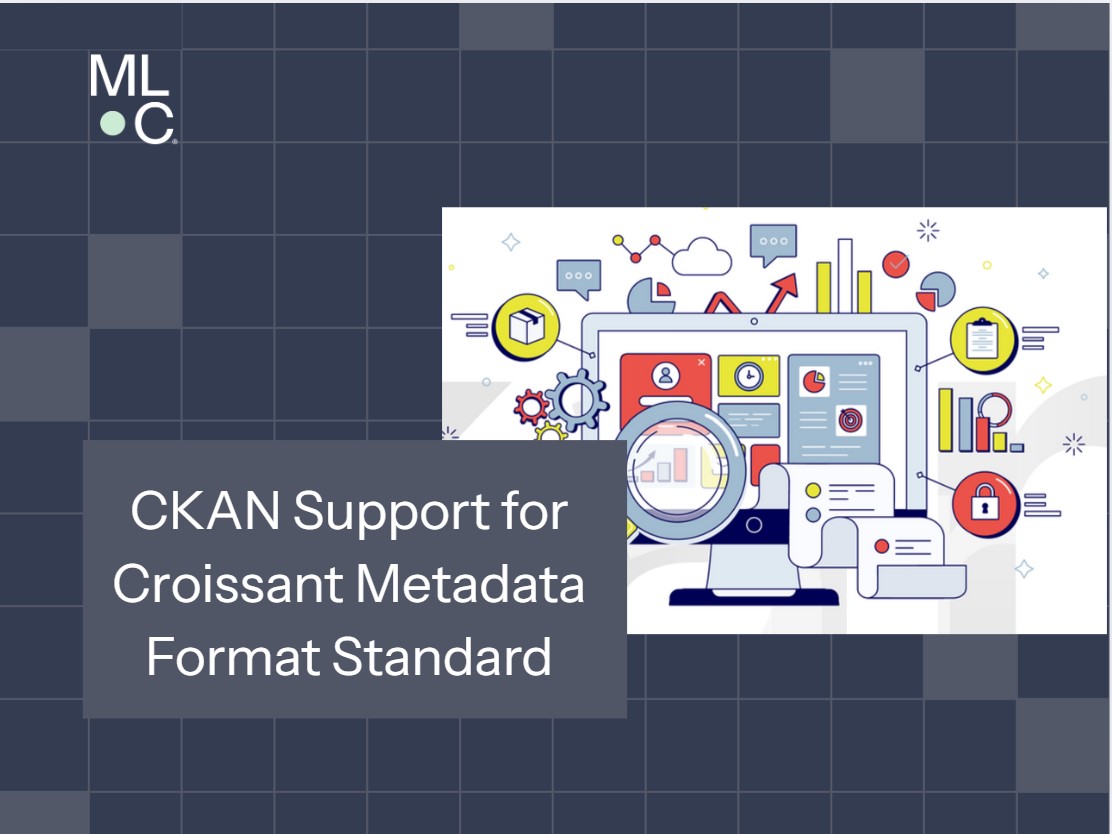
Datasets
Create new datasets to fuel innovation in machine learning.
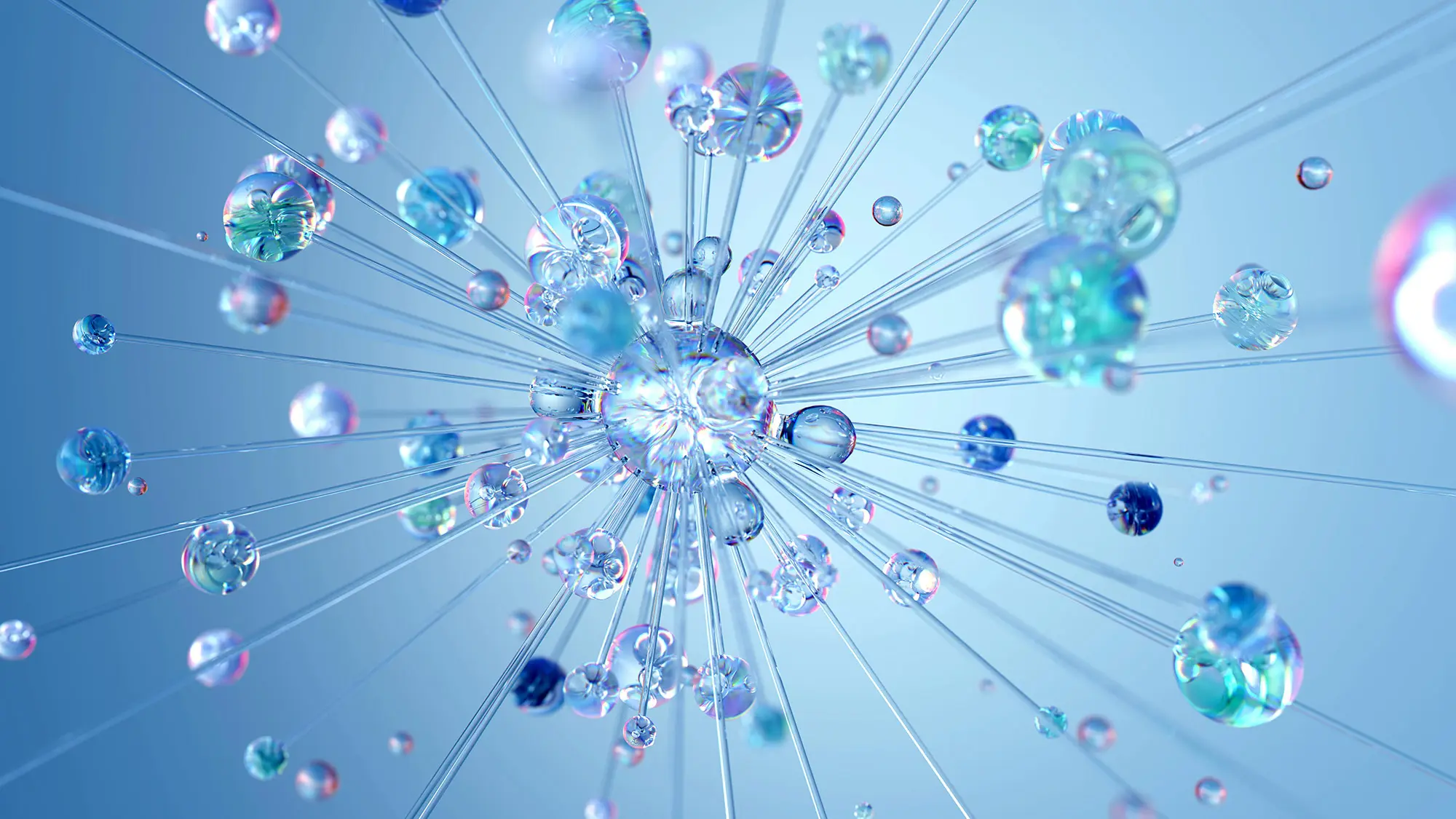
Purpose
Datasets fuel machine learning: a model is only as good as the data it was trained upon. ImageNet, created for less than half a million dollars, arguably gave rise to modern machine learning. Unfortunately, most public datasets today are either small (relative to private commercial datasets), static, licensed for research use only, or some combination of those things. Datasets must be large to train accurate models. To stay relevant, datasets should be constantly improved as gaps in their coverage are identified. Lastly, datasets require a permissive public license to enable new businesses, products, and services globally.
The Datasets working group creates and hosts public datasets that are large, actively maintained, and permissively licensed – especially for commercial use. We aim to develop a center of expertise and supporting technologies that dramatically improves the quality and reduces the cost of new public datasets. We believe that a modest investment in public datasets can have impressive ROI in terms of machine learning innovation and market growth. The Datasets Working Group’s first project was the People’s Speech dataset, an open speech recognition dataset that is approximately 100x larger than existing open alternatives.
Deliverables
- The People’s Speech Dataset v0.5 (100k hours of diverse speech)
- The People’s Speech Dataset v1.0 (100k hours of speech in 1,000 languages)
Meeting Schedule
Thursday May 8, 2025 Weekly – 11:05 – 12:00 Pacific Time
MLCommons Datasets
How to Join and Access Datasets Resources
To sign up for the group mailing list, receive the meeting invite, and access shared documents and meeting minutes:
- Fill out our subscription form and indicate that you’d like to join the Medical Working Group.
- Associate a Google account with your organizational email address.
- Once you’ve joined the Datasets Working Group, you’ll be able to access the Datasets folder in the Public Google Drive.
To engage in group discussions, join the group’s channels on the MLCommons Discord server.
To access the GitHub repositories (public):
- If you want to contribute code, please submit your GitHub ID to our subscription form.
- Visit the GitHub repositories:
Datasets Working Group Chairs
To contact all Datasets working group chairs email [email protected].