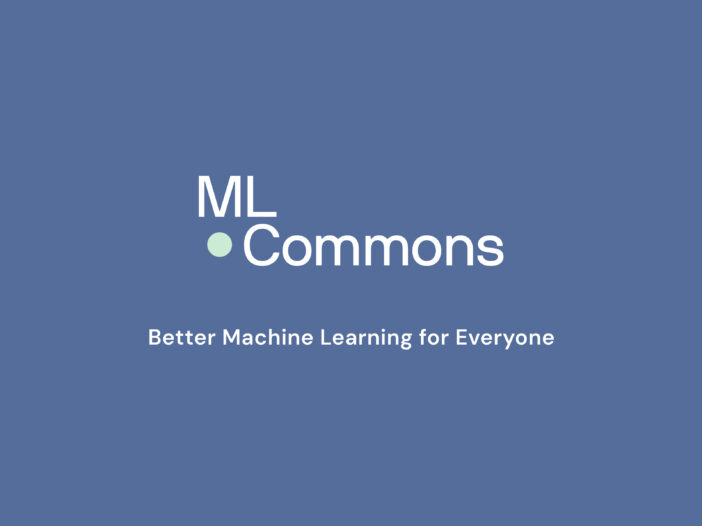
Today the MLPerf™ consortium released results for MLPerf HPC Training v0.7, the first round of results from their machine learning training performance benchmark suite for high-performance computing (HPC). MLPerf is a consortium of over 70 companies and researchers from leading universities, and the MLPerf HPC benchmark suite is establishing an industry standard for measuring machine learning performance on large-scale high performance computing systems.
The MLPerf HPC benchmark suite measures the time it takes to train emerging scientific machine learning models to a standard quality target in tasks relevant to climate analytics and cosmology. Both benchmarks make use of large scientific simulations to generate training data.
The first version of MLPerf HPC includes two new benchmarks:
- CosmoFlow: A 3D convolutional architecture trained on N-body cosmological simulation data to predict four cosmological parameter targets.
- DeepCAM: A convolutional encoder-decoder segmentation architecture trained on CAM5+TECA climate simulation data to identify extreme weather phenomena such as atmospheric rivers and tropical cyclones.
The MLPerf HPC Benchmark Suite was created to capture characteristics of emerging machine learning workloads on HPC systems such as large scale model training on scientific datasets. The models and data used by the HPC suite differ from the canonical MLPerf training benchmarks in significant ways. For instance, CosmoFlow is trained on volumetric (3D) data, rather than the 2D data commonly employed in training image classifiers. Similarly, DeepCAM is trained on images with 768 x 1152 pixels and 16 channels, which is substantially larger than standard vision datasets like ImageNet. Both benchmarks have massive datasets – 8.8 TB in the case of DeepCAM and 5.1 TB for Cosmoflow – introducing significant I/O challenges that expose storage and interconnect performance. The rules for MLPerf HPC v0.7 follow very closely the MLPerf Training v0.7 rules with only a couple of adjustments. For instance, to capture the complexity of large-scale data movement experience for HPC systems, all data staging from parallel file systems into accelerated and/or on-node storage systems must be included in the measured runtime.
“Our first set of results were submitted by organizations from around the world with a diverse set of HPC systems, demonstrating the enthusiasm in the HPC communities for supporting these emerging machine learning workloads,” said Steven Farrell (NERSC) of the latest release. “They also showcase the state-of-the-art capabilities of supercomputers for training large scale scientific problems, utilizing data-parallel and model-parallel training techniques on thousands to tens of thousands of processors.”
To see the results, go to mlcommons.org/en/training-hpc-07/.
The initial round saw submissions from the following organizations:
- Swiss National Supercomputing Centre (CSCS) – Led by Lukas Drescher and Andreas Fink
- Fujitsu – Led by Koichi Shirahata and Tsuguchika Tabaru at Fujitsu Laboratories
- Lawrence Berkeley National Laboratory (LBNL) – Led by Steven Farrell
- National Center of Supercomputer Applications (NCSA) – Led by Dawei Mu
- Japan’s Institute of Physical and Chemical Research (RIKEN) – Led by Aleksandr Drozd and Kento Sato
- Texas Advanced Computer Center (TACC) – Led by Amit Ruhela
MLPerf is committed to providing benchmarks that reflect the needs of machine learning customers at national labs and compute centers, and is pioneering the construction of benchmarks relevant to large scale data-driven machine learning for science. Jacob Balma (HPE) concluded, “These are future-oriented benchmarks aimed at measuring capabilities of modern supercomputers for these emerging workloads. This important step makes it possible to engineer future systems optimized for the next generation of machine learning algorithms.”
Additional information about the HPC Training v0.7 benchmarks will be available at mlcommons.org/en/training-hpc-07/.