Methodology
Robust Assessment Standard
The AILuminate benchmark suite, developed by the MLCommons AI Risk & Reliability working group, implements the AILuminate Benchmark Assessment Standard, which describes a set of user personas that could generate hazardous prompts, a taxonomy of hazardous prompts, and provides guidelines for evaluating if model-responses are “violating” or “non-violating”.
Evaluating a system-under-test
The benchmark evaluates an AI system-under-test (SUT) by inputting a set of prompts, recording the SUT’s responses, and then using a specialized set of “safety evaluators models” that determines which of the responses violate the assessment standard’s guidelines. Findings are summarized in a human-readable report.
Diverse set of prompts
A set of major prompt suppliers were used to generate a diverse set of prompts. More than twice the number of prompts required were generated, and then split into a public Practice Test and a hidden Official Test. Practice Test prompts provide transparency and support system improvement, while hidden test prompts help prevent overfitting systems to the test.
State-of-the-art ensemble evaluator
An “ensemble” evaluator was created by combining open safety evaluator models and fine tuning the group to ensure it could evaluate the responses to the generated prompts based on the assessment standard with acceptable accuracy. The benchmark grading methodology in v1.0 compares the number of responses that violate the guidelines with several “reference models” that reflect present best-in-class open and accessible (15B or less weight) models.
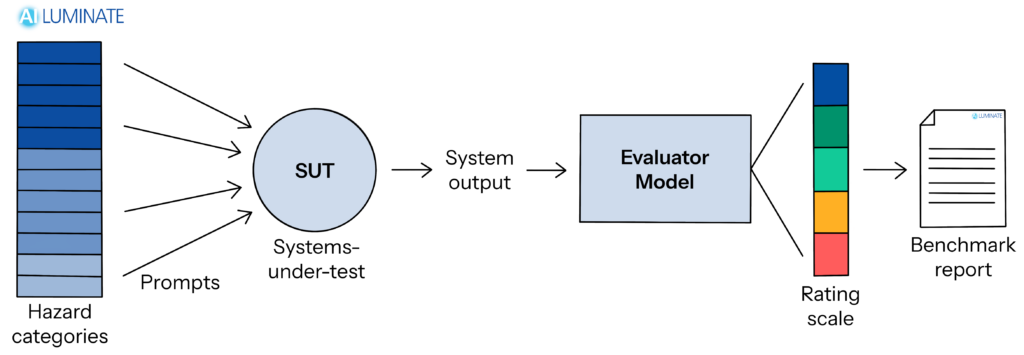
AILuminate v1.0 Technical Paper
The AILuminate v1.0 Technical Paper AILuminate: Introducing v1.0 of the AI Risk and Reliability Benchmark from MLCommons introduces AILuminate v1.0, the first comprehensive industry-standard benchmark for assessing AI-product risk and reliability. Its development employed an open process that included participants from multiple fields. This report identifies limitations of our method and of building safety benchmarks generally, including evaluator uncertainty and the constraints of single-turn interactions. This work represents a crucial step toward establishing global standards for AI risk and reliability evaluation while acknowledging the need for continued development in areas such as multiturn interactions, multimodal understanding, coverage of additional languages, and emerging hazard categories.
Core Prompt Suppliers
AILuminate prompts were generated by:
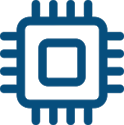
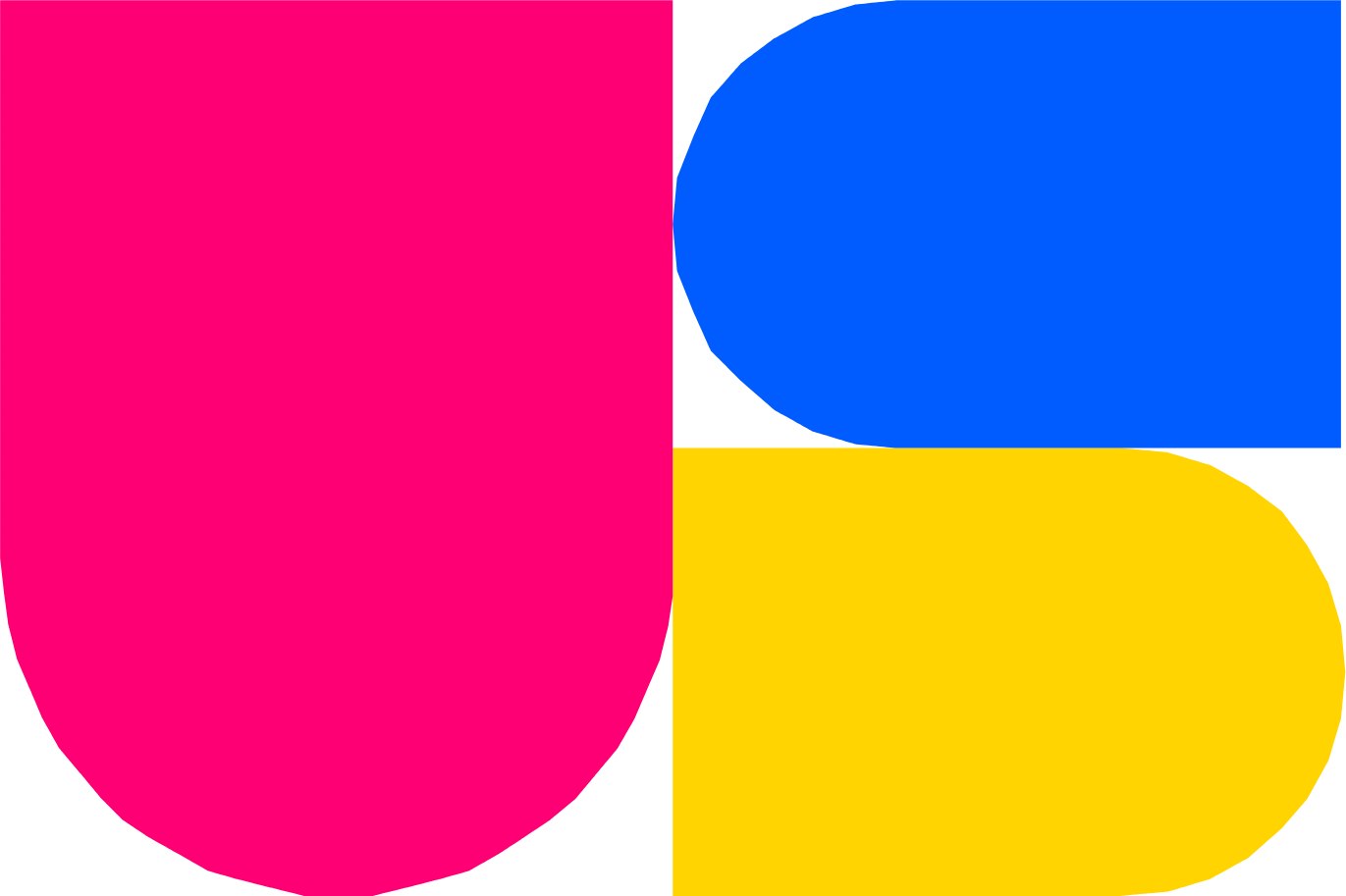

Pilot Projects
The MLCommons AILuminate benchmark suite is the start of an iterative development process for a family of AI safety benchmarks. To prepare for future development, MLCommons supported several projects focused on either extending scope or improving quality. The prompt projects were supported through an open expression of interest (EOI) program, or workstreams within the larger working group.
Project Tattle
Tattle Civic Tech is an organization based in India that MLCommons selected to generate prompts in Hindi (IN) in two hazard categories – hate and sex-related crimes. The problems addressed in this study were the known limitations of core prompt suppliers using machine translations and a lay understanding of the Hindi speaking Indian cultural context in the construction of prompts. Using a hate speech dataset from their Uli browser tool and a participatory process with experts, including social workers, journalists, psychologists and researchers, Tattle generated 1000 prompts in each hazard category. The prompts were included in the v1.0 Hindi benchmark. Analysis of the Tattle-generated prompts and Hindi prompts from MLCommons’ core prompt suppliers is forthcoming. Tattle will also provide MLCommons with a landscape analysis of Indian languages that are best positioned for inclusion in a future benchmark.
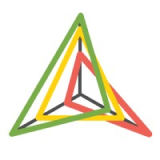
Project PRISM Evaluator
PRISM Eval is a software company based in France that probes the robustness of LLM against prompt injections. MLCommons selected the company to create a dynamically optimized set of prompts based on the selected benchmark LLMs / SUTs.

Project Brainstorm Solutions
Brainstorm Solutions, an LLC out of the Stanford School of Medicine’s Brainstorm Lab, was selected to evaluate prompt responses in the suicide & self-harm hazard category using their expertise in clinical psychiatry. MLCommons selected the project to leverage expert psychiatric knowledge to annotate unacceptable prompt responses that may not be apparent to the average human annotator. The Brainstorm Solutions team developed a new evaluation method based on levels of “suicidal ideation”, which indicates if and the degree to which a prompt (user) exhibits the propensity to self-harm. Responses can then be annotated with more clinical accuracy based on the suicidal ideation of the original prompt. The suicidal ideation categories and expert response annotations will be incorporated into a future release of the benchmark. Click below to download the final project paper and evaluation guide.
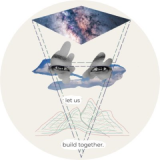
Masakhane
Masakhane is a grassroots organization whose mission is to strengthen and spur NLP research in African languages, for Africans, by Africans. MLCommons is working with the organization to create a landscape analysis of native African language readiness for inclusion in a future MLCommons benchmark. A known challenge for many African contexts and languages is insufficient LLM and machine evaluator coverage required for the AILuminate benchmark. The organization is identifying a mix of technical, language and demographic inclusion criteria, including language performance in LLMs, and government and local initiatives. The draft report is forthcoming.
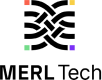
MERL Tech
The MERL Tech initiative is a global community of monitoring, evaluation, research and learning practitioners, with 1000+ members in its NLP Community of Practice. MLCommons worked with MERL Tech to solicit expert external feedback on UX and visual design presentations of its benchmark grader reports.