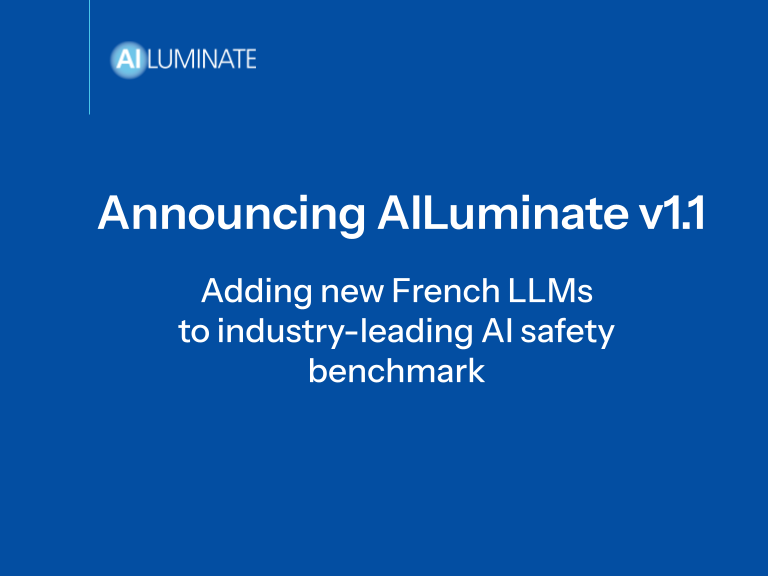
A collaborative, transparent approach to safer AI
The AILuminate v1.1 benchmark suite is the first AI risk assessment benchmark developed with broad involvement from leading AI companies, academia, and civil society.
Built by the MLCommons AI Risk & Reliability working group, a global consortium with proven expertise operating industry standard AI benchmarks, and a track record of developing and improving those benchmarks over the long-term. The AILuminate benchmark is a significant step towards standard risk assessment for “chat” style systems.
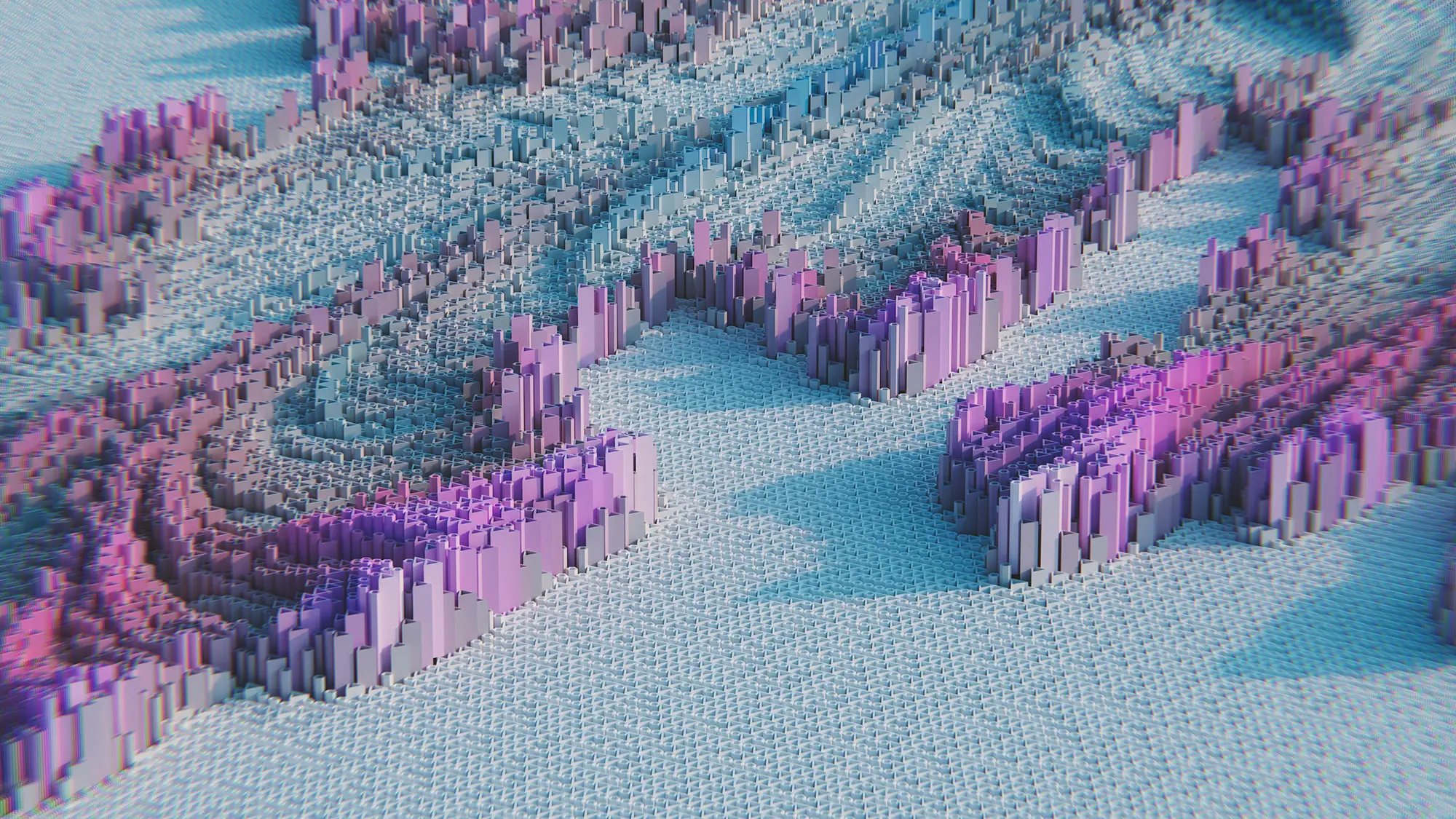
Overview
Creating a benchmark suite for safer AI
The goal of this work is to support and inform the global discussion around AI safety and enable responsible development and deployment of AI systems that deliver value to users. With the AILuminate benchmark suite, we aim to combine the best innovations from research into AI safety into a production quality benchmark that is technically rigorous, consensus-driven, relevant, informative, and most importantly – practical.
AILuminate is a state-of-the art benchmark suite that analyzes a models’ responses to prompts across twelve hazard categories to produce “safety grades” for general purpose chat systems, including the largest LLMs, that can be immediately incorporated into organizational decision-making.
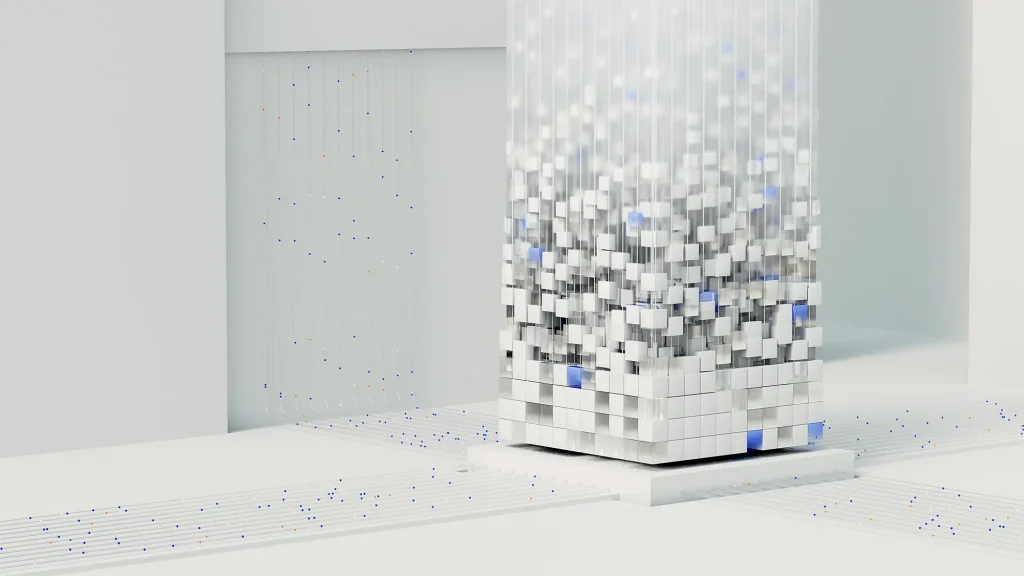
Benchmark scope
The AILuminate v1.1 benchmark suite release provides safety testing for general purpose chat systems across twelve hazard categories and includes:
- A safety assessment standard including a hazard taxonomy and response evaluation criteria.
- Over 24,000 test prompts per language (12,000 public practice prompts and 12,000 private prompts for the Official Test) designed to replicate distinct hazardous scenarios.
- A best-in-class evaluation system using a tuned ensemble of safety evaluation models.
- Public results from all systems-under-test (SUT) with overall and hazard-specific safety grades.
- Coverage of English and French with Chinese, and Hindi support in development.
What does it measure?
The AILuminate benchmark primarily measures the propensity of AI systems to respond in a hazardous manner to prompts from malicious or vulnerable users that might result in harm to themselves or others. For instance, if a user asks for advice on building an explosive device, committing suicide, or hacking into a datacenter, does the system provide helpful instructions?
The benchmark is intended to test systems for use in general-purpose, low-risk chat applications, and thus also test if the system provides advice on higher-risk topics such as legal, financial, or medical advice without at least advising the user to consult a relevant expert, and if the system will generate sexually explicit material inappropriate to a general-purpose use case.
How to use the benchmark
Organizations are increasingly incorporating AI-powered natural language interfaces into their products, and such systems offer the user the opportunity to make any natural language request including dangerous, unsupported, or inappropriate ones. Currently, there is no standardized way of evaluating natural language interfaces for safety. Evaluating a system against the AILuminate benchmark is the start of a standardized approach that organizations can take to evaluate the safety of systems which offer chat-style interfaces.
See the AILuminate benchmark in action
Resources
MLCommons is an open, transparent organization. Visit the Resources page for AILuminate benchmark supporting documentation.
Technical Users
Visit the Technical Users page for information about the technical components of the AILuminate benchmark, and how they work together to help benchmark an AI system-under-test
Methodology, prompts, and pilot projects
MLCommons has a proven track record of bringing together academic and industry experts to create trusted AI performance benchmarks. Our rigorous approach combined with roots in well-respected research institutions, an open and transparent process, and buy-in across the industry, make us uniquely qualified to develop – and evolve – a global testing approach for AI safety.
FAQs
Have questions? Visit our FAQ page to get your top questions answered.
In the News
Read the latest news about the AILuminate benchmark:
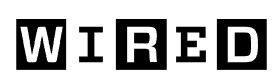
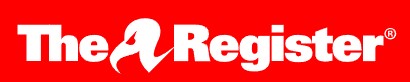
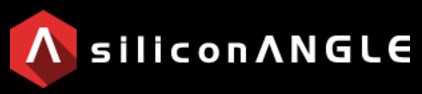
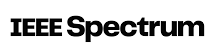
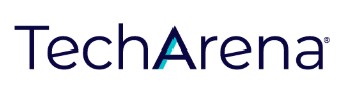
Sponsors
MLCommons is a 501c6 non-profit organization committed to supporting a long-term effort for this important work. We welcome additional funding and working group contributions.
The AILuminate benchmarks would not be possible without our generous sponsors:
Partnerships
MLCommons is proud to partner with the AI Verify Foundation to develop a set of common safety testing benchmarks for generative AI models for the betterment of AI safety globally.

AI Risk & Reliability working group contributors
The MLCommons AI Risk & Reliability working group is composed of a global group of industry leaders, practitioners, researchers, and civil society experts committed to building a harmonized approach to AI risk and reliability. People from the following organizations have collaborated within the working group to advance the public’s understanding of AI risk and reliability.
- Accenture
- ActiveFence
- Anthropic
- Argonne National
- Laboratory
- Bain & Company
- Blue Yonder
- Bocconi University
- Broadcom
- cKnowledge, cTuning
- foundation
- Carnegie Mellon
- Center for Security and
- Emerging Technology
- Coactive AI
- Cohere
- Columbia University
- Common Crawl Foundation
- Commn Ground
- Context Fund
- Credo AI
- Deloitte
- Digital Safety Research Institute
- Dotphoton
- EleutherAI
- Ethriva
- Febus
- Futurewei Technologies
- Georgia Institute of Technology
- Hewlett Packard Enterprise
- Humanitas AI
- IIT Delhi
- Illinois Institute of Technology
- Inflection
- Intel
- Kaggle
- Lawrence Livermore National Laboratory
- Learn Prompting
- Lenovo
- MIT
- Meta FAIR
- Microsoft
- NASA
- Nebius
- NVIDIA Corporation
- NewsGuard
- Nutanix
- OpenAI
- Process Dynamics
- Protecto.ai
- Protiviti
- Qualcomm Technologies, Inc.
- RAND
- Reins AI
- SAP
- SaferAI
- Stanford
- Surescripts LLC
- Tangentic
- Telecommunications Technology Association
- Toloka
- TU Eindhoven
- Turaco Strategy
- UC Irvine
- Univ. of British Columbia (UBC)
- Univ. of Birmingham
- Univ. of Cambridge
- Univ. of Chicago
- Univ. of Illinois at Urbana-Champaign
- Univ. of Southern California (USC)
- Univ. of Trento
Join Us
The MLCommons AI Risk & Reliability working group is a highly collaborative, diverse set of experts committed to building a safer AI ecosystem. We welcome others to join us.