The MLCommons® Medical working group recently presented a “Federated Learning in Healthcare” tutorial at IEEE International Symposium on Biomedical Imaging (ISBI) 2024. The tutorial provided participants an introduction and hands-on experience to the Comprehensive Open Federated Ecosystem (COFE). COFE is an open ecosystem of tools and best practices distilled from years of industrial and academic research experience. It aims to streamline the research and development of AI/ML models within the clinical setting.
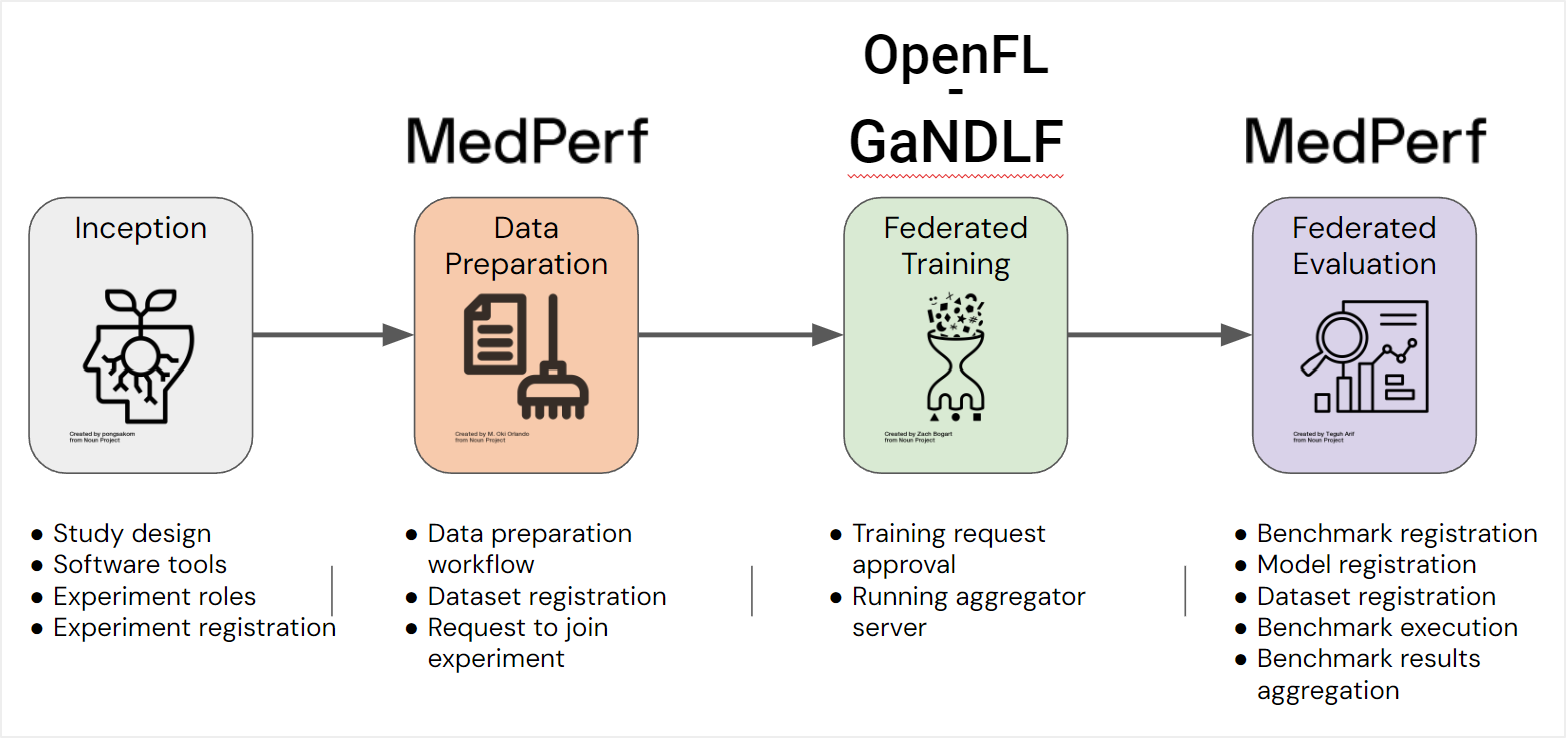
An AI-enabled clinical study under COFE. Each step involves actions carried out in the tools offered within COFE
In a nutshell, COFE consists of the following tools working seamlessly:
- The Generally Nuanced Deep Learning Framework (GaNDLF) – https://gandlf.org/
- MedPerf – https://medperf.org/
- OpenFL – https://openfl.io/
A fundamental principle of COFE is its federated approach which enables researchers to train and evaluate AI across multiple institutions without sharing their sensitive patient data. In this way COFE facilitates a clinical study on real-world data in a more efficient and secure way.
During the tutorial session Spyridon (Spyros) Bakas, MLCommons Medical working group’s vice chair for benchmarking & clinical translation and director of the Center for Federated Learning in Medicine and for the Computational Pathology Division at Indiana University, introduced attendees to the benefits of federated AI by enabling large global efforts such as FeTS, and for advancing generalization of AI on diverse datasets.
Running federated AI experiments requires simplicity and efficiency given the technical complexities introduced by multi-institute participation. Sarthak Pati, software architect at Indiana University & technical lead at (MLCommons, shared new insights on how to easily train and design custom models using MLCommons’ GaNDLF in real-world settings. Specifically, attendees were presented with examples of how GaNDLF provides an easy to use solution to create end to end clinical workflows for training and deploying AI solutions across multiple problem domains (e.g., segmentation, classification, and regression).
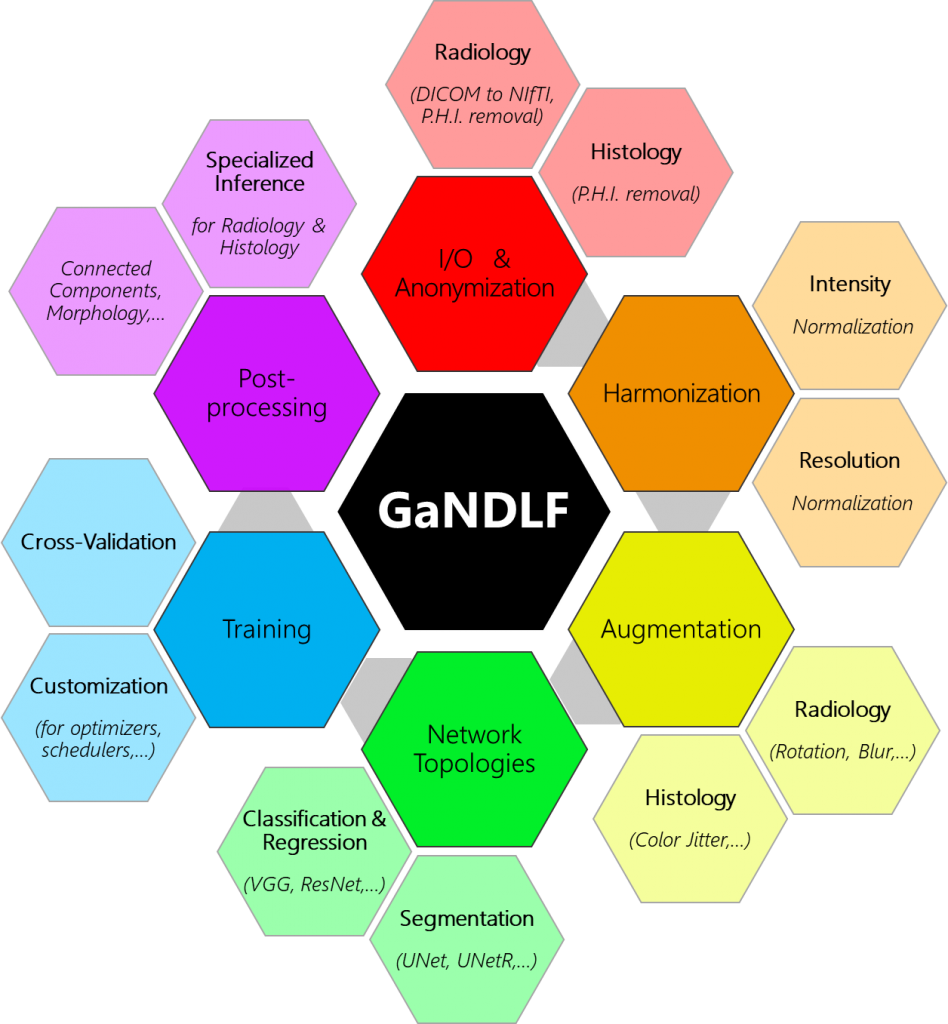
Overview of computational tasks available with GaNDLF
To address smooth data preparation and efficient orchestration and monitoring of federated AI experiments, MedPerf was introduced at Nature Machine Intelligence in 2022. Hasan Kassem, ML engineer at MLCommons, demonstrated through hands-on examples how MedPerf simplifies data preparation and experient orchestration in a real-world clinical study through hands-on examples. To accomplish this, MedPerf is built on a simple architecture relying on transparent actions performed via key roles and responsibilities of stakeholders in a medical AI experiment.
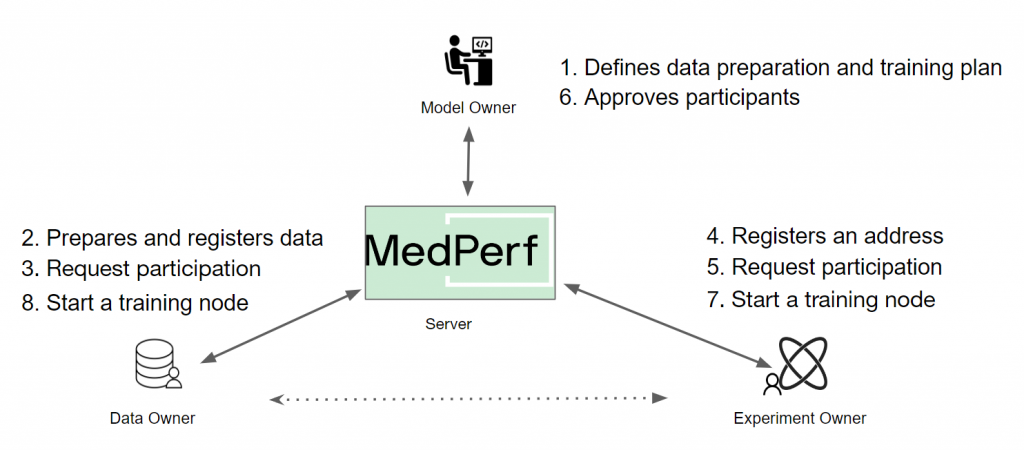
Orchestration of a federated AI experiment using MedPerf
Siddhesh Thakur, data engineer at Indiana University School of Medicine, showcased optimization workflows and their practical application in deploying AI models for whole slide image analysis in resource-constrained environments. Thakur’s presentation explored key concepts of model optimization, demonstrating how GaNDLF facilitates this process by integrating with Intel’s OpenVINO toolkit. Through online examples, attendees learned how to leverage these tools to create efficient, deployable AI solutions for digital pathology, even in settings with limited computational resources.
When it comes to federated AI experiments a key step is training AI models in a federated learning fashion. Federated learning can take place in various topologies (vertical vs horizontal) adding technical complexity in real world federations, such as data splits. Meanwhile securing the process is paramount for federated settings, increasing further complexity. Intel’s OpenFL, hosted by The Linux Foundation, is a flexible, extensible and easily learnable tool, designed for data scientists, that aims to build federated learning settings to address these issues. Walter Riviera, AI technical lead at Intel and Ph.D. candidate at University of Verona, demonstrated running federated learning on various topologies, and shared the challenges of implementing FL across both axis. In particular, new challenges with data, model, hardware, and frameworks architecture heterogeneity reflect the need for the research community to continue to expand on the utility of aggregation functions, improve security, and explore better ways to enhance communication speed.
The Federated Learning in Healthcare tutorial was made possible through GitHub Codespaces with generous technical support from their engineers and program managers working in close collaboration with the tutorial organizers. More information about the tutorial can be found here.
We would also like to thank the organizing committee of ISBI 2024 and in particular the conference organizers: Konstantina S. Nikita Conference. Christos Davatzikos, Spyretta Golemati, and Elisa E. Konofagou.
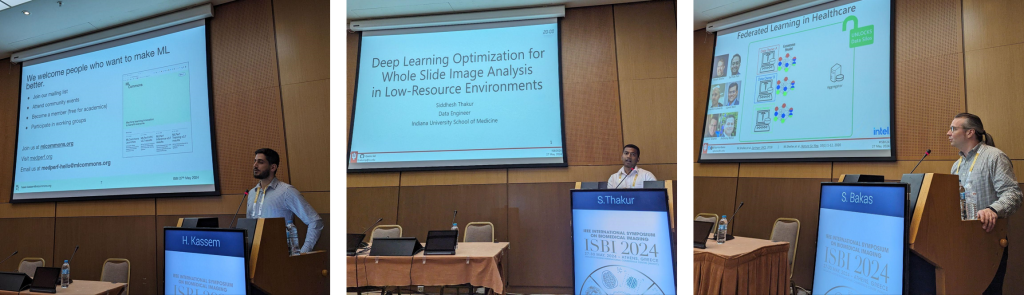
Federated healthcare AI experts presenting components of COFE in Athens at ISBI 2024. From left to right: Hasan Kasem presented MedPerf, a federated evaluation platform to run benchmarks of medical AI models in real-world data securely and privately. Siddhesh Thakur presented an example of federated learning in digital pathology in low-resource environments using GaNDLF. Spyridon Bakas introduced the audience to the importance of federated datasets in healthcare as well as federated learning concepts
Join Us
COFE addresses key challenges that the research community faces in the federated healthcare AI space through open community tools. As an organization we strive to collect feedback from the broader healthcare research community to help build tools that advance AI/ML for everyone. If you are interested in participating in the MLCommons Medical working group please join here.