By Sarthak Pati, Alexandros Karargyris, Spyridon Bakas
Today we are excited to share the publication of the Generally Nuanced Deep Learning Framework (GaNDLF) in the Nature Communications Engineering journal. This is a major milestone for the MLCommons® Medical Working Group, as GaNDLF describes the outcome of a global collaboration by distinct research groups spanning industry and academia. GaNDLF is a powerful framework following zero/low code principles and hence alleviating programming requirements for medical researchers to easily take advantage of the latest AI developments and analyze healthcare data. It enables analysis of multiple data types (e.g., radiology, histology) and tasks (e.g., segmentation, classification), while being agnostic to anatomies and pathologies. GaNDLF is the first step towards creating a standardized mechanism to define end-to-end healthcare AI applications into larger clinical workflows.
Creating a General-Purpose Framework for Healthcare AI
GaNDLF was inspired by our work on the Federated Tumor Segmentation (FeTS) initiative. We realized that we needed a general-purpose framework for healthcare AI that could define end-to-end clinical workflows for various healthcare use-cases such as tumor segmentation and molecular classification. To address this need, we built GaNDLF, which is the first healthcare-focused framework that can handle multiple types of data and AI workloads (see Figure 1).
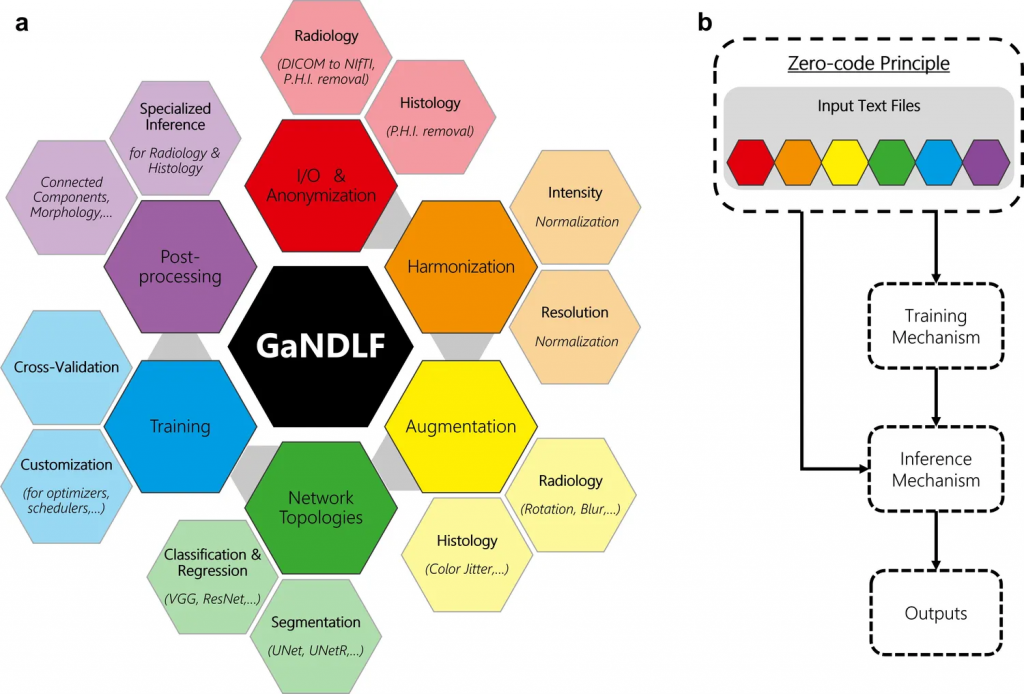
Figure 1: Current amalgamation of the functionality of GaNDLF.
A Zero/Low Code Approach
There is tremendous potential for AI to solve problems in healthcare such as tumor segmentation, but adoption in a clinical setting is very challenging. And while clinical researchers have a deep understanding of different problems in healthcare, they often lack expertise in AI and may not be able to design robust solutions.
GaNDLF addresses this issue through a zero/low code approach to help clinical researchers focus-in on the healthcare challenges and abstract away the complexity of AI tools. For example, using GaNDLF, a medical researcher can efficiently train a model without writing a single line of code. GaNDLF performs automatic post-training optimization so trained models do not require any specialized hardware during inference, easing deployment. Additionally, GaNDLF seamlessly integrates with OpenFL, enabling researchers to easily use federated learning across multiple institutions and patient populations, greatly increasing the quality and diversity of data for training and evaluation and ultimately leading to better and more robust treatments.
Faster Prototyping and Baseline Results
GaNDLF’s focus on zero/low code principles and state-of-the-art algorithms enables fast prototyping and generation of baseline results in a fraction of the time of traditional methods. The ability to quickly and easily deploy trained models on a variety of host systems without requiring specialized hardware ensures that models trained using GaNDLF are usable in low resource environments. GaNDLF has been extensively tested and validated to ensure its accuracy, and the team is confident that it will quickly become an essential tool in medical research and practice.
Call for Participation in the GaNDLF Community-Driven Effort
Open, inclusive, collaborative efforts, such as GaNDLF, can drive innovation and bridge the gap between AI research and real-world clinical impact. To achieve these benefits, there is a critical need for broad collaboration, reproducible, standardized and open computation, and a passionate community that spans academia, industry, and clinical practice. We welcome additional participation and look forward to your joining us.
We call for the following:
- Clinical researchers interested in training AI models without having to write a single line of code. GaNDLF enables them to generate baseline results for their data in a quick and reproducible manner. Additionally, models trained using GaNDLF do not require any specialized hardware for deployment.
- Computational researchers interested in increasing the applicability and reach of their algorithms. By developing their method on GaNDLF, it ensures their method(s) can be applied across different data types and application domains in healthcare.
- Group Leaders in academia who want to ensure reproducibility and continuity of research. GaNDLF provides a common integration API for different computational and processing pipelines, ensuring that clinical workflows can be maintained after individual researchers leave the group.
- Anyone interested in researching federated learning studies can leverage GaNDLF’s seamless integration with Linux Foundation’s OpenFL and MLCommons’ MedPerf to facilitate no-code design of federations.
We would like to thank our co-authors of the Nature Communications Engineering article for their valuable contributions throughout the development and research phase of GaNDLF. This effort would not be possible without the generous support from members of the following organizations (in alphabetical order): Dana-Farber, Google, Intel, MLCommons, University of Edinburgh, and University of Pennsylvania. For more information, please read the Nature Communications Engineering paper and consider joining the MLCommons Medical working group to contribute to our open-source efforts, including GaNDLF.